Data Science is a sufficiently ambiguous term to become part of a jargon stew kept warm by the repeated vague assertions that it is the solution to every problem ailing businesses, non-profits, governments, families, and possibly nature. And yet, its interest hasn't fallen off the hype cycle like so many buzzwords before. Why?
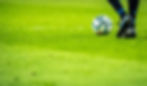
Consider a professional organization as a soccer team. As a field, Data Science often a middling soccer mid-fielder with sometimes amazing plays. Left to its own devices it sometimes delivers brilliantly! This justifies continuing the use of data science, even when pinning down what it is can be difficult. The road to solid great Data Science outcomes can be problematic. Gartner expects that the next several years to have an 85% failure rate in data science projects stemming from this situation exactly -- leaving a great team player to figure out their own biases, strengths, competencies, and use to the overall team. This is despite 70% of executives believing analytics to be "very important" while only 2% believing they have seen "broad positive results" (as reported by The Economist).
So -- why be satisfied with a sometimes amazing 2% success rate? To continue the sports idea, you want to make sure the team you're coaching performs well together. Understanding the cycle of data science helps you ensure performance and delivery of your data science efforts integrate with your other activities and operations for improved performance.

The data science cycle is key to unlocking investment value in digital services. The elements of the cycle are
Solution formation: as an organization, you have a problem that you formulate a process to resolve. Perhaps it is in operations, in sales, in research and strategy, or other groups building the path to success. At this stage, the solution is typically heavily manual and takes a lot of time.
Digitization: components of the solution begin to be committed to software. It could be the entire process or parts of it
Monitoring: as digitization evolves, data capture for the process begins. Data models and infrastructure support are set up, and software continues to improve and integrate.
Analytics: data capture opens the door for solution owners and stakeholders to begin to ask concrete questions about the nature and implementation of the process that require data to answer. User engagement, trends, "what-if" scenario and forecast questions, and more.
Data science: methodologies, tools, and approaches to answer the questions analytics raises. Identifies areas of further development to manage uncertainty in processes (turning the digital services model into a feedback cycle). Highest value-add in the value chain. Greatly enhances the continuous improvement processes of software by incorporating data-driven responses and issue identification in existing solution processes.
Can digital services exist without the Data Science cycle? Certainly! Just like manual solutions that haven't adopted the data science cycle of data-driven improvement, these digital services rely on the assumptions, hopes, and hunches of the solution designers and software developers to achieve acceptable delivery. Improvement of the processes are qualitative, hopeful, and often expensive. The solution owners and stakeholders might have no need to understand how their solution behaves and performs, and don't need a competitive edge to improve their organizations.
For those that want to put their organizations onto cycles of data-driven continuous improvement, we at Flamelit are committed to improving your world too through data science development and delivery.